Q-Cloud Use Cases
Use Case: High-Throughput
Take advantage of Q-Cloud’s high flexibility. The size of a Q-Cloud server is highly customizable, and scales up or down automatically based on usage. Reduce costs by paying only for the compute nodes you actually use.
Customer A commercial company developing organic LEDs (OLEDs) wants to use DFT and TDDFT to screen several thousand possible OLED molecules, with the goal of selecting a small subset of candidates that are likely to perform most efficiently based on their singlet-triplet gaps. They have a team of computational chemists who use a handful of on-site workstations or small on-premises cluster, but they do not have access to a large supercomputing cluster.
Problem/Opportunity This high-throughput screening process could provide significant cost reduction in terms of manpower within the lab setting by allowing researchers to focus on a limited set of synthetic candidates. However, while the screening is infrequent, it requires significant computational power to provide results in a timely manner. Purchasing and maintaining a hundred-node cluster for on-premises use would be wasteful and incur significant costs, given that most of the nodes on that cluster will spend a significant amount of time idle.
Solution Using Q-Cloud, this user can run a high volume of Q-Cloud calculations in parallel in the cloud. The user can utilize up to 100 nodes at once, and they only pay for their monthly Q-Cloud license fee and the AWS computational resources they need to run their jobs. When the calculations are done, the additional nodes are released, and the size of the Q-Cloud cluster reduces back to just the head node.
Use Case: High-Performance
Accurately simulating large or complicated molecules often requires access to expensive high-performance computing (HPC) architecture, even if only a few jobs are run at once. Q-Cloud on AWS offers HPC nodes on-demand, making these types of simulations accessible to companies without their own HPC cluster.
Customer A smaller commercial company is focusing on the development of transition metal complexes for CO2 activation. Although the company does not have a dedicated theory team, a small number of employees have a hybrid background in both experiment and computational chemistry, and they want to take full advantage of their skill set by supplementing their experimental research and development with theory.
Problem/Opportunity Advanced post-HF methods, such as EOM-CC, can be used alongside useful analysis tools (such as EDA) to provide significant insights about reaction mechanisms, binding energies, and other useful physical properties. These calculations can help researchers better understand the systems they work with, leading to more efficient product development. However, sufficiently-accurate simulations of large transition metal complexes can incur steep computational costs, requiring many compute hours and consuming significant amounts of memory. Purchasing and maintaining high-performance computing (HPC) systems capable of handling these jobs would require a significant investment of both money and labor. Because the team has no way to demonstrate the cost savings associated with this approach before investing in the hardware, this move will likely be considered high-risk.
Solution This company could purchase a low-volume Q-Cloud license that allows them to run up to 10 jobs at once. This provides their small team of researchers with the HPC infrastructure they need to run their calculations, without the need for investing in expensive on-site hardware.
Use Case: Flexible Licensing Model
For some users, a software as a service (SaaS) provides an appealing alternative to a permanent licensing model. Reduce start-up costs. Maintain your access to the most up-to-date version, and cancel if you decide that the service is no longer required.
Customer A start-up company is interested in running quantum chemistry calculations. They will have a small team of theoreticians to run calculations, but they have not yet invested in substantial on-site computing infrastructure. Minimizing initial start-up costs is a top priority.
Problem/Opportunity This customer has a significant opportunity to use simulations to aid in their research, but there are two problems stopping them from doing so: Up-front costs, and scaling. Firstly, because the company is trying to minimize their start-up costs, paying the one-time cost of a permanent Q-Chem license up front is not ideal. Additionally, because they are a new company, they may not yet have a solid idea of the scale or number of calculations they will be running. They could request a demo license, but that would require purchasing and maintaining their own on-premises hardware, incurring more start-up costs. If they buy too much hardware, they risk being wasteful; if they don’t buy enough, they will limit their team’s ability to produce results efficiently.
Solution Q-Cloud’s subscription-based licensing model is a great choice for this customer. It avoids the steep initial costs associated with obtaining a permanent license, and it ensures that the company always has access to all of the most recent features. For new companies, or those who are just beginning to explore the world of computational chemistry, Q-Cloud also provides an appealing option in terms of flexibility. If the researchers find that they are not using Q-Cloud as often as anticipated, they can simply reduce or cancel the subscription before the beginning of the next billing cycle. Conversely, if the researchers find that they need to run more jobs than they initially thought, they can easily increase their license entitlement.
Use Case: Academic Group
A young academic has just established a research group and wants to model the reaction mechanisms associated with novel synthetic pathways to drug candidates. The group would like to test a range of levels of theory to determine which of them model the systems most accurately.
Problem/Opportunity Due to rising electricity costs and increasing overheads of running computing resources, the university is decommissioning their central computing resources. Furthermore, the research group does not have the in-house expertise required to purchase, set up and maintain a small-scale cluster for the group. This group would like to maintain their current research trajectory without the need for on-site computing resources.
Solution The group leader can purchase a Q-Cloud license with appropriate capacity for their initial group size and easily expand the cluster later on as the group size grows. Additionally, they can create the cluster in a region where running costs are lower, thus avoiding the local electricity prices. Furthermore, students can access Q-Cloud easily from anywhere, with no need for a university VPN. Q-Cloud’s installer allows fast, easy setup of Q-Cloud on AWS hardware. The hardware on the cluster is highly customizable and can be upgraded or altered as needed, and the cluster administrator can use AWS’s CPU monitoring and cost management tools to track the number of compute hours the group is spending or set automatic notifications whenever certain cost thresholds are reached.
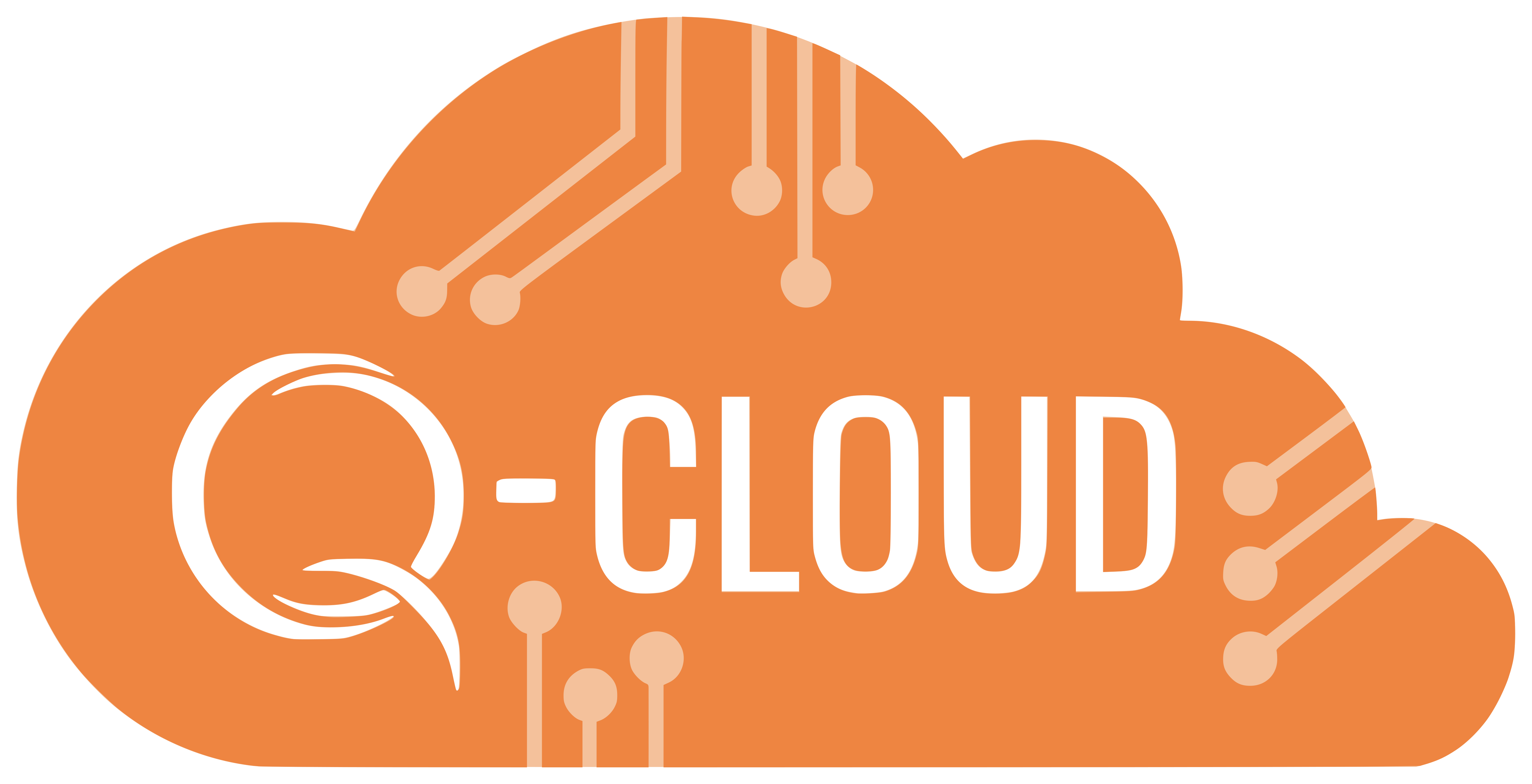